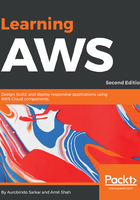
Deploying cloud-based machine learning models
The model scoring environments can be very diverse. For example, models may need to be deployed in web applications, portals, real-time and batch processing systems, as an API or a REST service, embedded in devices or in large legacy environments.
The technology stack can comprise Java Enterprise, C/C++, legacy mainframe environments, relational databases, and so on. Additionally, non-functional requirements and customer SLAs with respect to response times, throughput, availability and uptime can also vary widely. However, in all cases our cloud deployment process will need to support A/B testing, experimentation, model performance evaluation, and be agile and responsive to business needs.
Typically, practitioners use various methods to benchmark and phase-in new or updated models to avoid high-risk big bang production deployments. We will explore more on deploying such applications in Chapter 10, Deploying a Big Data Application.